Wisdom of the crowd? Building better forecasts from suboptimal predictors
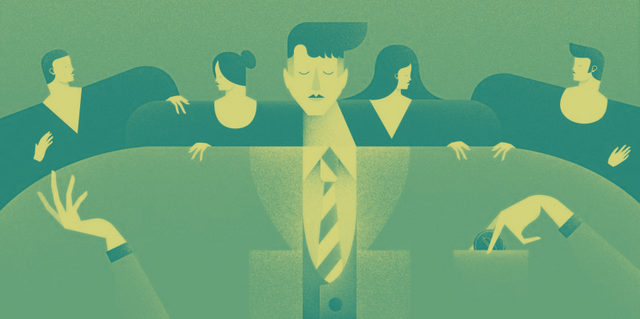
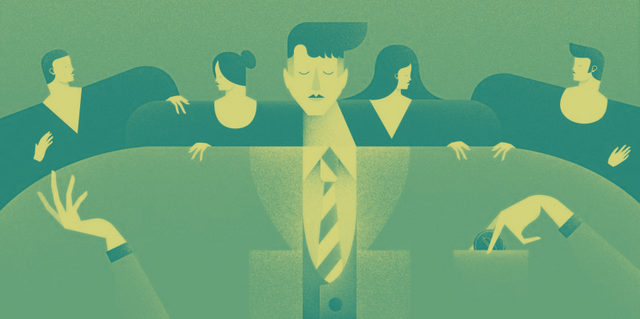
Researchers at the University of Tokyo and Kozo Keikaku Engineering Inc. have introduced a method for enhancing the power of existing algorithms to forecast the future of unknown time series. By combining the predictions of many suboptimal forecasts, they were able to construct a consensus prediction that tended to outperform existing methods. This research may help provide early warnings for floods, economic shocks, or changes in the weather.
Time series data are a familiar part of our daily lives. A gyrating graph might represent the water level of a river, the price of a stock, or the daily high temperature in a city, just to name a few. Advance knowledge of the future movements of a time series could be used to avert or prepare for future undesirable events.
However, forecasting is extremely difficult because the underlying dynamics that generates the values are nonlinear (even if assumed to be deterministic) and therefore subject to wild fluctuations.
Delay embedding is a widely used method to help make sense of time series data and attempt to predict future values. This approach takes a sequence of observations and "embeds" them in a higher-dimensional space by combining the current value with evenly spaced lagged values from the past.
For example, to create a three-dimensional delay embedding of the S&P 500 closing price, you can take the closing prices today, yesterday, and the day before as the x-, y-, and z-coordinates, respectively. However, the possible choices for embedding dimension and delay lag make finding the most useful representation for making forecasts a matter of trial and error.
Now, researchers at the University of Tokyo and Kozo Keikaku Engineering Inc. have showed a way to select and optimize a collection of delay embeddings so that their combined forecast does better than any individual predictor. "We found that the 'wisdom of the crowd,' in which the consensus prediction is better than each on its own, can be true even with mathematical models," first author Shunya Okuno explains.
The researchers tested their method on real-world flood data, as well as theoretical equations with chaotic behavior. "We expect that this approach will find many practical applications in forecasting time series data, and reinvigorate the use of delay embeddings," senior author Yoshito Hirata says.
Forecasting a future system state is an important task in many different fields including neuroscience, ecology, finance, fluid dynamics, weather, and disaster prevention, hence this work has potential for use in a wide range of applications.
Story Source: Materials provided by Institute of Industrial Science, The University of Tokyo. Note: Content may be edited for style and length.
Journal Reference: Shunya Okuno, Kazuyuki Aihara, Yoshito Hirata. Forecasting high-dimensional dynamics exploiting suboptimal embeddings. Scientific Reports, 2020; 10 (1) DOI: 10.1038/s41598-019-57255-4
Posted from my blog with SteemPress : https://www.psycupdates.com/wisdom-of-the-crowd-building-better-forecasts-from-suboptimal-predictors/
Hi! I am a robot. I just upvoted you! I found similar content that readers might be interested in:
https://www.sciencedaily.com/releases/2020/01/200120113430.htm